The goal of BL.Formation
Each year, territorial agents can claim training courses and their managers can also ask for trainings for the department. In medium to large municipalities, these requests are sent to the training managers in the HR department. There can be a significant amount of training requests to compile and classify. Training managers group similar requests together to easily form training groups (intra or union), in order to optimize their training resources. This grouping process is a repetitive task with no real added value for training managers. Automating these tasks can allow training managers to focus on budgetary trade-offs, specific agents’ needs, and additional information needed to build and follow the annual training plan. This automation can be achieved through artificial intelligence.
The aim of this Master 2 project in Human-Computer Interaction is to design and develop an interface to inspect, manipulate and supervise the results of an AI clustering the training requests. The main concerns are to ensure that automation is a benefit and not a burden for the user, and that he keeps confidence in the AI results.
Ensuring the Human-AI collaboration
The field of Human-Computer Interaction aims to understand the capabilities of humans and systems to find the best possible interaction between them. We therefore addressed the following question: How to ensure collaboration between the Human (training manager) and the AI (clustering algorithm)? This issue guided our user-centered design work, and the communication with the DRIT AI experts to best use existing technologies. This reflection with stakeholders led us to design various concepts of data visualization and screen space management (Figure 1) for improving Human-AI collaboration.
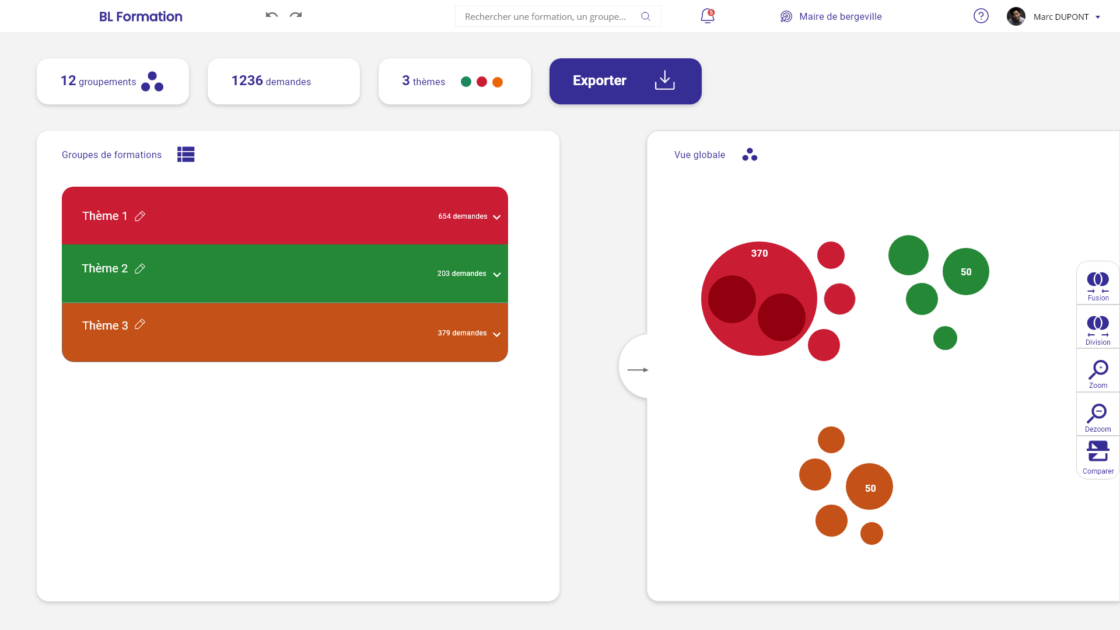
Such an interface allows the user to visualize the results of the AI: on the left side he can consult the details of the clusters in a “tree view” (opened in Figure 2), and on the right side he can have an overview of all the clusters in a “circle packing” visualization. The clusters are organized by themes (color) which name is freely editable by the user.
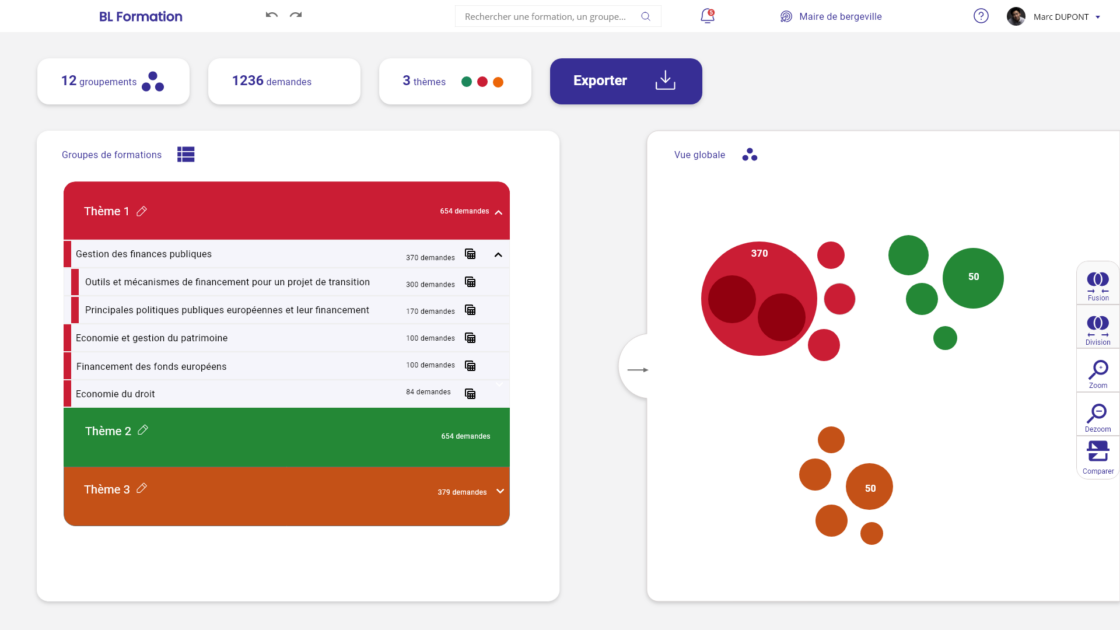
The user can consult the details of a training cluster in a spreadsheet view (Figure 3) by clicking on the spreadsheet icon. The collapsible mini-map can always be displayed to see all the clusters at any time and manipulate them through the toolbar.
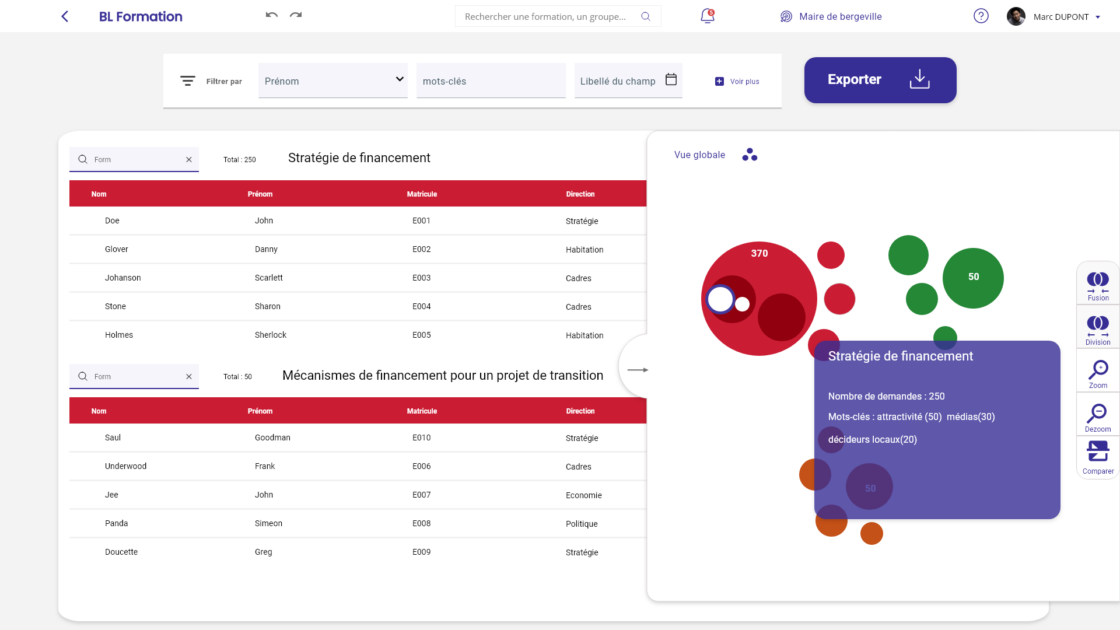
To improve the clusters proposed by the IA, the user can:
- Compare the details of two clusters (Figure 4)
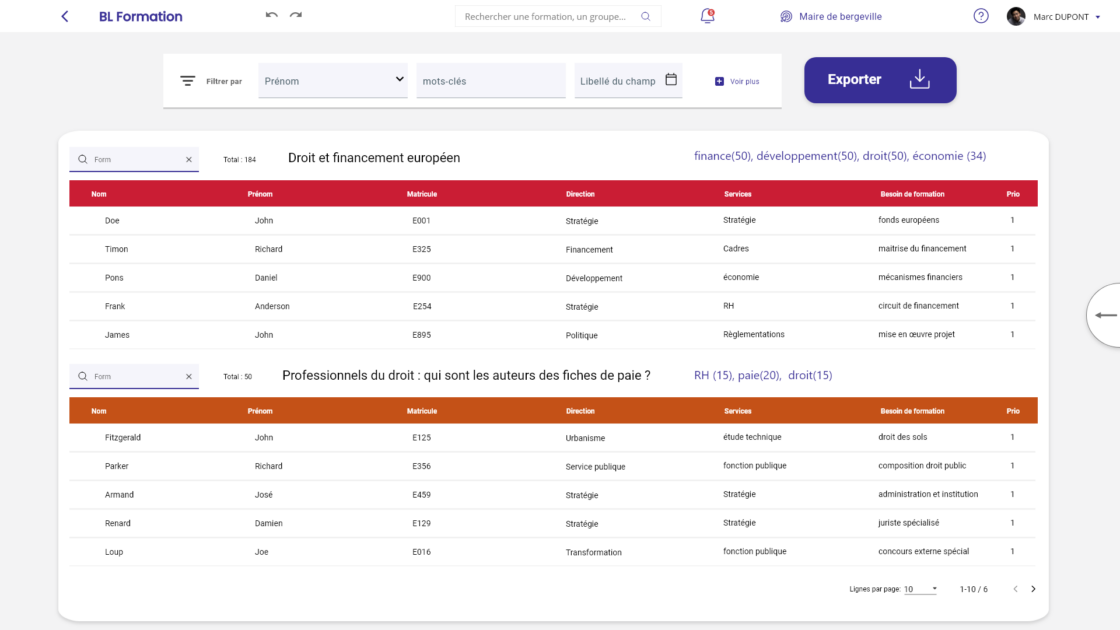
- Merge two clusters, if they are too similar (Figure 5)
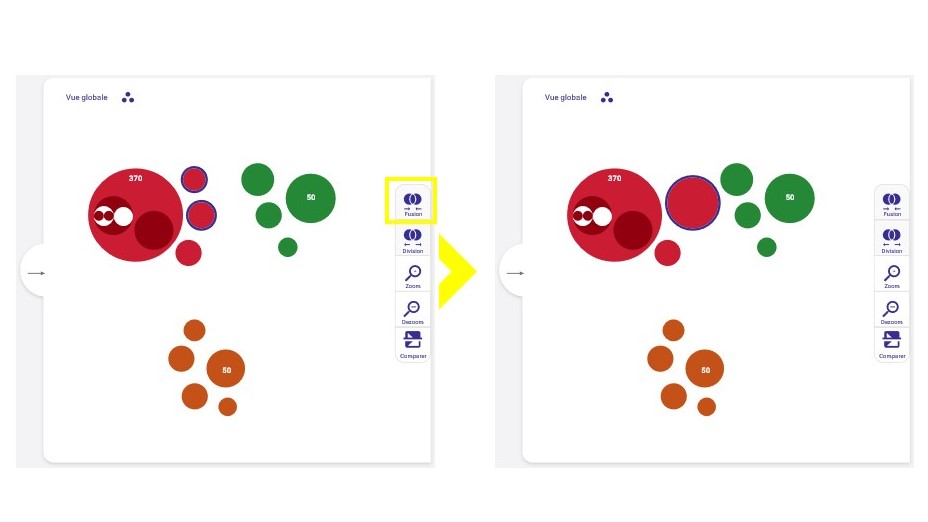
- Ask the AI to divide a cluster into several sub-groups, if the number of requests in one group is too large or too diversified (Figure 6)
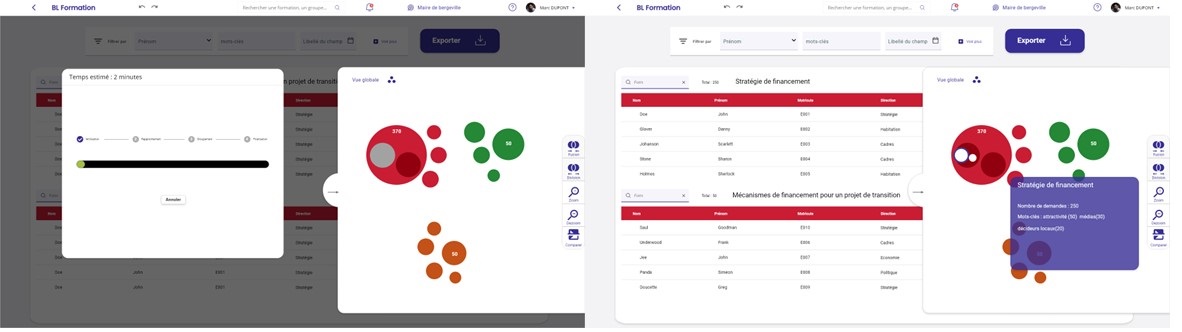
At any time, the user can export the content of his choice, whether it is a single cluster or all the clusters, in an Excel file to constitute the annual training plan.
Added value of BL.Formation
Training request files can be thousands of lines long, BL.Formation saves the manager the trouble of going through the entire request file and filtering the titles to find similar titles over and over again. The application eases the management of the training clusters for several agents and thus significantly saves time in the managers’ work process.
Thanks to the interactivity of the data visualization and the ability to directly modify the results, human-IA collaboration is very fluid. The complex AI algorithm can process a large amount of data and facilitate the work of the professional, as it is well integrated into his usual work process.
Conclusion
The mission of BL.Formation is to facilitate the aggregation of similar training requests for the constitution of the annual training plan of municipalities. These repetitive tasks are automated by an AI algorithm. Supervision and control of the user over the AI is established through BL.Formation interface which allows to observe, manipulate, and modify the clusters thus constituted.
For now, BL.Formation is a standalone prototype, but it is intended to become part of BL. Assistant, a marketplace of interactive intelligent robots. They will help city managers in all their daily tasks that require a balance between automation and some level of human expertise.